March 30, 2007 —
The predictive learning, which is a core issue of the DRIVSCO project, needs to be tested and evaluated.
For this purpose a robot setup was established at BCCN. It consists of a commercially available robot
platform (Volksbot) that has been modified to meet the special needs of the application.
For instance, a mounting platform was added that allows installing two cameras for stereo vision,
and provides space for a laptop. Different tyres were chosen for reducing friction, etc.
The robot is shown in fig. 1. To model a real world scenario more realistically, the robot is embedded
into a sensory-action-loop, where its recorded images are displayed on a TV observable by a human,
who in turn can remote control the robot by manipulating a game steering and pedal set.
Fig. 1. Robot setup (Click here to see the video-1,24 MB).
A control architecture which comprises of predictive/sequence learning algorithms must be evaluated.
To prevent the test car from damage a robot setup was established. In addition from sparing the car
it provides the opportunity to easily create certain situations that might be more difficult to obtain
in a real world scenario, and also to repeat them under the same conditions (illumination, weather, traffic).
Because in a real world scenario a human is driving the car, the same must be assured for the robot setup.
This issue was solved by adding a game steering wheel and pedal set, which can be manipulated by a driver to remote
control the robot. In addition the driver is supposed to only dispose of the visual information acquired by the robot.
Therefore it was arranged that the camera images from the robot are displayed on a TV that is situated in front of
the human. A schematic drawing of this architecture is shown in fig. 2, and a real world example in fig.3.
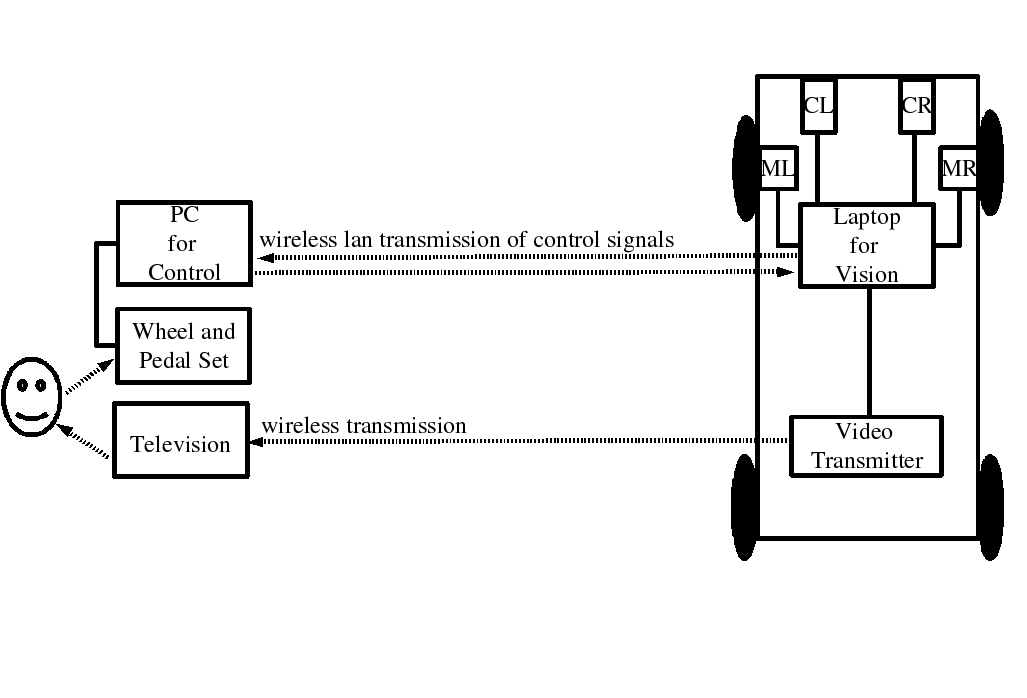
Fig. 2. Schematic drawing of the architecture of the robot.
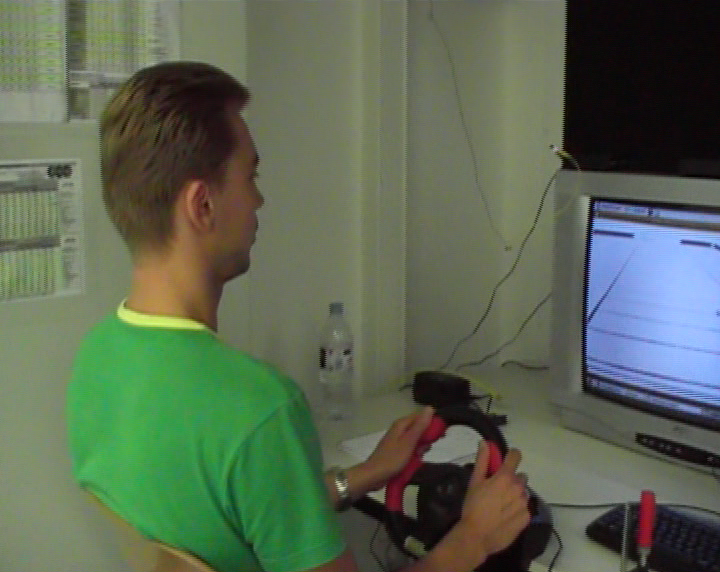
Fig. 1. Real world example.
Action plan:
The first goal that the robot system is to achieve is to learn to follow the street.
Based on this, more complex behaviours shall be added to the repertoire, such as reacting to street signs
and handling unforeseen situations like sudden obstacles.
To follow the street the robot must be equipped with means to recognize the features necessary for this task.
Therefore a street lane extraction tool was implemented which processes each camera frame. The obtained mathematical
representation (cubic splines) of the lanes will be used for a predictive road following learning routine.
For constant speed it was already possible to show that the robot can learn from the driver to autonomously
follow the street. A video can be viewed.
Hardware facts:
The used cameras are from AVT type Marlin F 080C and comprise of a IEEE 1394 (firewire) interface. The chip is a Sony IT CCD with a diagonal of 1/3'', (6 mm). With these cameras a transfer rate of 20/30 Hz can be achieved where the image resolution is 1032 x 778, depending on which kind of image data is acquired (raw or color images).
The used lenses are Fujinon vari-focal lenses (2.7-13.5mm), which at the lowest focal length allow a horizontal opening angle of approx. 90 degrees, where a radial distortion (straight lines appear curved, an effect that gets stronger towards the outer limits of the picture.
Concerning the robot there is a fundamental difference to a car, i.e. its axes are fixed. Thus, steering is realized by slowing down the wheels on one side and accelerating the ones on the other.
Irene Markelic, Tomas Kulvicius, Florentin Woergoetter (1)
Norbert Krueger (2)
Minija Tamosiunaite (3)
(1) Department of Computational Neuroscience, Georg-August-University Göttingen;
(2) The Maersk Mc-Kinney Moller Institute, University of Southern Denmark;
(3) Department of Applied Informatics, Vytautas Magnus University.
|